CuMiDa
CuMiDa
An Extensively Curated Microarray DatabaseOne might have notice a pattern, when applying machine learning techniques in cancer microarray datasets: they are scattered through multiple repositories, normally from old studies, being employed time and time again for the same purposes. However, the reality is that the microarray technology has changed, from their chip technology and number of known probes to their preprocessing options. Hence, continuing employing the same examples and old datasets, already manipulated by older studies, is not in agreement with the reality we have nowadays. Right now, microarray datasets contain more genes, come from multiple platforms and need a more rigorous filtering and preprocessing to be ready for machine learning approaches.
Here we present the Curated Microarray Database (CuMiDa), a repository containing 78 handpicked cancer microarray datasets, extensively curated from 30.000 studies from the Gene Expression Omnibus (GEO), solely for machine learning. The aim of CuMiDa is to offer homogeneous and state-of-the-art biological preprocessing of these datasets, together with numerous 3-fold cross validation benchmark results to propel machine learning studies focused on cancer research. The database make available various download options to be employed by other programs, as well for PCA and t-SNE results. CuMiDa stands different from existing databases for offering newer datasets, manually and carefully curated, from samples quality, unwanted probes, background correction and normalization, to create a more reliable source of data for computational research.
How to Cite
If you use CuMiDa in a scientific publication, we would appreciate citations to the following paper:
BibTeX
@article{cumida:2019,
author = {Feltes, B.C. and Chandelier, E. B. and Grisci, B. I. and Dorn, M.},
title = {CuMiDa: An Extensively Curated Microarray Database for Benchmarking and Testing of Machine Learning Approaches in Cancer Research},
journal = {Journal of Computational Biology},
volume = {26},
number = {4},
pages = {376-386},
year = {2019},
doi = {10.1089/cmb.2018.0238}
}
Workflow
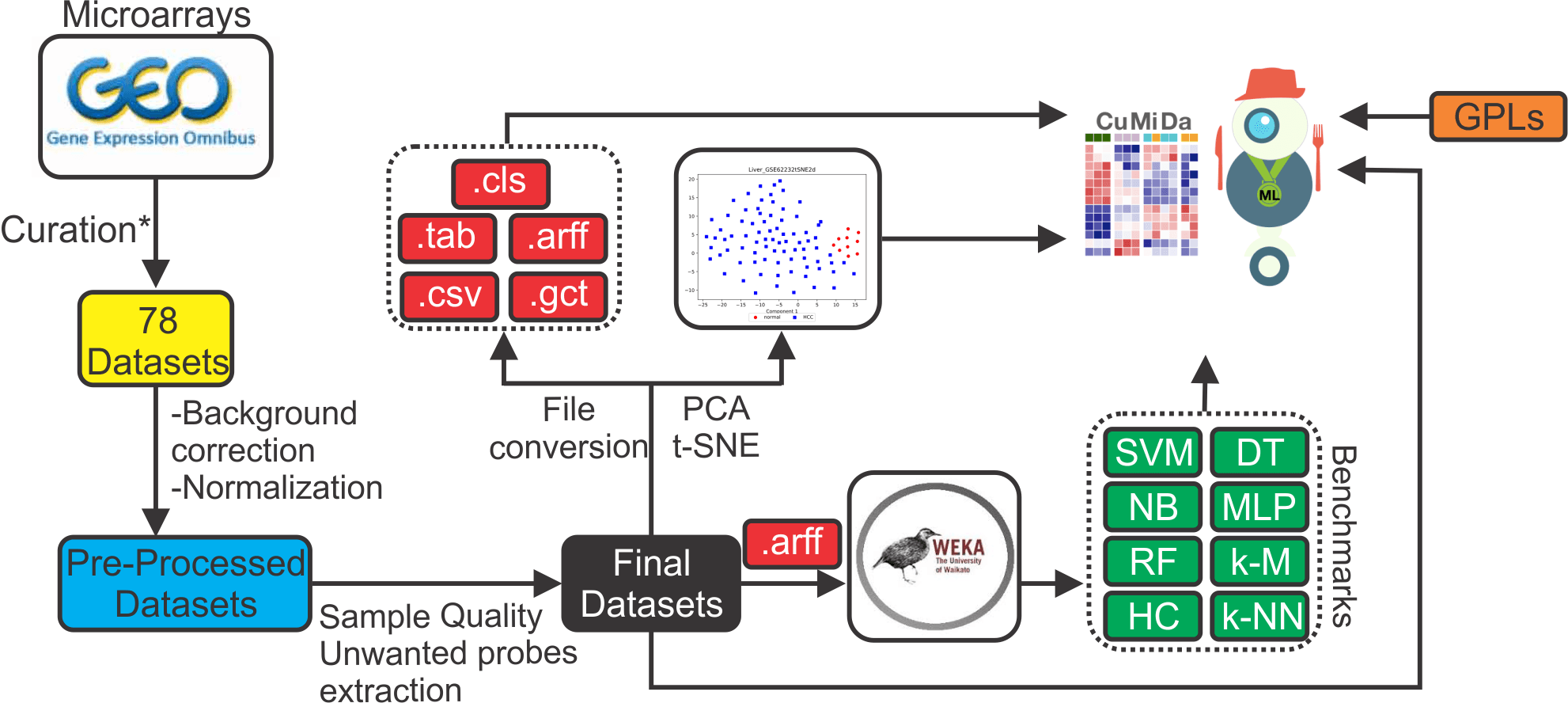