Research Areas
Computer Science: Heuristic Search Methods
Metaheuristics combine basic heuristic methods in a higher-level framework aimed at efficiently and effectively exploring a search space that guides the search for a solution in a broad range of optimization problems. The main goal is to find an acceptable solution with an acceptable time. Most of the metaheuristics consist of interaction with local improvements (exploitation) and strategies that avoid being trapped in local optima (exploration).
In Bioinformatics there are several problems that still do not have a computational method that can guarantee a minimum quality of solution in a feasible time. This is due to the fact that, the rules that govern the biochemical processes and relations are partially known, making harder to design efficient computational strategies. Since such problems are classified as NP-Complete or NP-Hard, there is the need to use computational techniques that can deal with them.
Metaheuristics are one of the most common and powerful techniques used in this case. They do not guarantee the optimal solution, but they give a good approximation with a limited computational effort.
Tools and Datasets
Science is rapidly advancing towards a paradigm of greater transparency and accessibility. This shift encompasses not only the sharing of data but also extends to publications, computer code, and methodologies.
In recent years, SBCB Lab has developed many tools, libraries, and datasets to foster collaboration and enhance accessibility within the scientific community. Our commitment to free access to these resources reflects our belief in the importance of leveling the playing field and empowering researchers from diverse backgrounds to contribute meaningfully to scientific progress.
Publications
2024
- Molecular Basis of MC1R Activation: Mutation-Induced Alterations in Structural Dynamics CAVATAO, F. G.; PINTO, E. S. M.; KRAUSE, M. J.; ALHO, C. S.; DORN, M. PROTEINS: Structure, Function, and Bioinformatics, v. 92, p. 1-15, 2024.
- Enhancing classification with hybrid feature selection: A multi-objective genetic algorithm for high-dimensional data BOHRER, J. S.; DORN, M. Expert Systems with Applications, v. 255, p. 124518, 2024.
- Sodium propionate oral supplementation ameliorates depressive-like behavior through gut microbiome and histone 3 epigenetic regulation BEHRENS, L. M. P.; GASPAROTTO, J.; RAMPELOTTO, P. H.; ESCALONA, M. A. R.; SILVA, L. S.; CARAZZA-KESSLER, F. G.; BARBOSA, C. P.; CAMPOS, M. S.; DORN, M.; GELAIN, D. P.; MOREIRA, J. C. F. The Journal of Nutritional Biochemistry, v. 130, p. 109660, 2024.
- Analysis and comparison of feature selection methods towards performance and stability BARBIERI, M. C.; GRISCI, B. I.; DORN, M. Expert Systems with Applications, v. 241, p. 1-30, 2024.
- Exploring bacterial diversity and antimicrobial resistance gene on a southern Brazilian swine farm TORRES, M. C.; BREYER, G. M.; ESCALONA, M. A. R.; MAYER, F. Q.; VARELA, A. P. M.; AZEVEDO, V. A. C.; DA COSTA, M. M.; ABURJAILE, F. G.; DORN, M.; BRENING, B.; CARDOSO, M. R. I.; SIQUEIRA, F. M. Environmental Pollution, v. 352, p. 124146, 2024.
- Just-in-Time Fluid Flow Simulation on Mobile Devices Using OpenVisFlow and OpenLB TEUTSCHER, D.; KUMMERLANDER, A.; BUKREEV, F.; DORN, M.; KRAUSE, M. J. Applied Sciences, v. 14, p. 1784, 2024.
- The MAPSTROKE project: A computational strategy to improve access to acute stroke care CARBONERA, L. A.; RIVILLAS, J. A.; PERUE, G. G.; DORNELES, L. L.; BOIANI, M.; SOUZA, A. C.; SILVA, G. S.; DORN, M.; MARTINS, S. C. O. International Journal of Stroke, 2024.
- Assessment of Kaistella jeonii esterase conformational dynamics in response to poly(ethylene terephthalate) binding PINTO, E. S. M.; MANGINI, A. T.; NOVO, L. C. C.; CAVATAO, F. G.; KRAUSE, M. J.; DORN, M. Current Research in Structural Biology, v. 7, p. 100130, 2024.
2023
- MC1R and age heteroclassification of face phenotypes in the Rio Grande do Sul population BETTIM, C. A.; VASCONCELLOS, A.; KAHMANN, A.; DORN, M.; SILVA, E. F. A.; ALHO, C. S. International Journal Of Legal Medicine, v. 138, p. 859–872, 2023.
- Functional response of microbial communities in lab-controlled oil-contaminated marine sediment JUNIOR, R. A.; POLONI, J. F.; ESCALONA, M. A. R.; DORN, M. Molecular Omics, v. 19, p. 756-768, 2023.
- Computational and integrative approaches for developmental biology and molecular evolution FELTES, B. C.; LIGABUE-BRAUIN, R.; DORN, M. Frontiers in Genetics, v. 14, p. 1252328, 2023.
- Meta-analyses of host metagenomes from colorectal cancer patients reveal strong relationship between colorectal cancer-associated species ESCALONA, M. A. R.; POLONI, J. F.; KRAUSE, M. J.; DORN, M. Molecular Omics, v. 19, p. 429-444, 2023.
- The nucleotide excision repair proteins through the lens of molecular dynamics simulations PINTO, E. S. M.; KRAUSE, M. J.; DORN, M.; FELTES, B. C. DNA Repair, v. 127, p. 103510, 2023.
- NIAS-Server 2.0: A versatile complementary tool for structural biology studies FELTES, B. C.; PINTO, E. S. M.; MANGINI, A. T.; DORN, M. Journal of computational Chemistry, v. 44, p. 1610-1623, 2023.
- An evolutionary algorithm based on parsimony for the multiobjective phylogenetic network inference problem VILLALOBOS-CID, M.; DORN, M.; CONTRERAS, A.; INOSTROZA-PONTA, M. Applied Soft Computing, v. 139, p. 110270, 2023.
- CBCovid19EC: A dataset complete blood count and PCR test for COVID-19 detection in Ecuadorian population ORDOÑEZ-AVILA, R.; PARRAGA-ALAVA, J.; HORMAZA, J. M.; VACA-CÁRDENAS, L.; PORTMANN, E.; TERÁN, L.; DORN, M. Data in Brief, v. 47, p. 109016, 2023.
- Implicit propagation of directly addressed grids in lattice Boltzmann methods KUMMERLÄNDER, A.; DORN, M.; FRANK, M.; KRAUSE, M. J. Concurrency and Computation: Practice and Experience, v. 35, p. 1-25, 2023.
- Ancestry resolution of South Brazilians by forensic 165 ancestry-informative SNPs panel FELKL, A. B.; AVILA, E.; GASTALDO, A. Z.; LINDHOLZ, C. G.; DORN, M.; ALHO, C. S. Forensic Science International Genetics, v. 64, p. 102838, 2023.
- Transcriptomic Analysis of Long Non-Coding RNA during Candida albicans Infection GONÇALVES, G. F.; POLONI, J. F.; DORN, M. Genes, v. 14, p. 251, 2023.
- The use of gene expression datasets in feature selection research: 20 years of inherent bias? GRISCI, B. I.; FELTES, B. C.; POLONI, J. F.; DORN, M. Wiley Interdisciplinary Reviews-Data Mining and Knowledge Discovery, v. 14, p. e1523, 2023.
- Interdisciplinary Overview of Lipopeptide and Protein-Containing Biosurfactants JÚNIOR, R. A.; POLONI, J. F.; PINTO, E. S. M.; DORN, M. Genes, v. 14, p. 76, 2023.
2022
- The Drosophila melanogaster ACE2 ortholog genes are differently expressed in obesity/diabetes and aging models: Implications for COVID-19 pathology DUARTE, T.; SILVA, M. M.; MICHELOTTI, P.; BARBOSA, N. B. V.; FELTES, B. C.; DORN, M.; ROCHA, J. B. T.; DALLA CORTE, C. L. Biochimica et Biophysica Acta (BBA) - Molecular Basis of Disease, v. 1868, p. 166551, 2022.
- Gene Expression Variation Analysis (GEVA): A new R package to evaluate variations in differential expression in multiple biological conditions NUNES, I. J. G.; FELTES, B. C.; DAVID, M. Z.; DORN, M. Journal of Biomedical Informatics, v. 129, p. 104053, 2022.
- Feature selection reveal peripheral blood parameter's changes between COVID-19 infections patients from Brazil and Ecuador FELTES, B. C.; VIEIRA, I. A.; PARRAGA-ALAVA, J.; MEZA, J.; PORTMANN, E.; TERÁN, L.; DORN, M. Infection, Genetics and Evolution, v. 98, p. 105228, 2022.
- Genetic and molecular Omp25 analyses from worldwide Brucella canis strains: Possible mutational influences in protein function LOPES, C. E.; DE CARLI, S.; FELTES, B. C.; PINTO, E. S. M.; SALA, R. D. V.; DORN, M.; SIQUEIRA, F. M. Gene, v. 817, p. 146175, 2022.
2021
- Would GENEALOMICS be an appropriate term to designate family tree research based on genome-wide data? ALHO, C. S.; DORN, M.; AVILA, E. Journal of Genetic Genealogy, v. 9, p. 91002, 2021.
- Transdisciplinary Approach for Bioinformatics Education in Southern Brazil DORN, M.; LIGABUE-BRAUN, M.; VERLI, H. Frontiers in Education, v. 6, p. 725591, 2021.
- Benchmarking and Testing Machine Learning Approaches with BARRA:CuRDa, a Curated RNA-Seq Database for Cancer Research FELTES, B. C.; POLONI, J. F.; DORN, M. Journal of Computational Biology, v. 28, p. 931-944, 2021.
- Comparison of machine learning techniques to handle imbalanced COVID-19 CBC datasets DORN, M.; GRISCI, B. I.; NARLOCH, P. H.; FELTES, B. C.; AVILA, E.; KAHMANN, A.; ALHO, S. C. PeerJ Computer Science, v. 7, 2021.
- Modifying the catalytic preference of alpha-amylase toward n-alkanes for bioremediation purposes using in silico strategies PINTO, E. S. M.; FELTES. B. C.; PEDEBOS, C.; DORN, M. Journal of Computational Chemistry, v. 42, p. 1540-1551, 2021.
- A Study on Shape-Dependent Settling of Single Particles with Equal Volume Using Surface Resolved Simulations TRUNK, R.; BRETL, C.; THÄTER, G.; NIRSCHL, H.; DORN, M.; KRAUSE, M. J. Computation, v. 9, p. 1-35, 2021.
- Evaluation of drug repositioning by molecular docking of pharmaceutical resources available in the Brazilian healthcare system against SARS-CoV-2 GRAHL, M. V. C.; ALCARÁ, A. L.; PERIN, A. P. A.; MORO, C. F.; PINTO, E. S. M.; FELTES, B. C.; GHILARDI, I. M.; RODRIGUES, F. V. F.; DORN, M.; COSTA, J. C.; SOUZE, O. N.; LIGABUE-BRAUN, R. Informatics in Medicine Unlocked, v. 23, p. 100539, 2021.
- Relevance aggregation for neural networks interpretability and knowledge discovery on tabular data GRISCI, B. I.; KRAUSE, M. J.; DORN, M. Information Sciences, v. 559, p. 111-129, 2021.
2020
- Multi-Approach Bioinformatics Analysis of Curated Omics Data Provides a Gene Expression Panorama for Multiple Cancer Types FELTES, B. C.; POLONI, J. F.; NUNES, I. J. G.; FARIA, S. S.; DORN, M. Frontiers in Genetics, v. 11, 2020.
- Hemogram data as a tool for decision-making in COVID-19 management: applications to resource scarcity scenarios AVILA, E.; KAHMANN, A.; ALHO, C. S.; DORN, M. PeerJ, v. 8, p. e9482, 2020.
- ConfID: an analytical method for conformational characterization of small molecules using molecular dynamics trajectories POLÊTO, M. D.; GRISCI, B. I.; DORN, M.; VERLI, H. Bioinformatics, v. 36, p. 3576-3577, 2020.
- Solving fluid flow domain identification problems with adjoint lattice Boltzmann methods KLEMENS, F.; FÖRSTER, B.; DORN, M.; THÄTER, G.; KRAUSE, M. J. Computers & Mathematics with Applications, v. 79, p. 17-33, 2020.
2019
- Integrated analysis of the critical region 5p15.3–p15.2 associated with cri-du-chat syndrome CORRÊA, T.; FELTES, B. C.; RIEGEL, M. Genetics and Molecular Biology, v. 42, p. 186-196, 2019.
- CuMiDa: An Extensively Curated Microarray Database for Benchmarking and Testing of Machine Learning Approaches in Cancer Research FELTES, B. C.; CHANDELIER, E. B.; GRISCI, B. I.; DORN, M. Journal of Computational Biology, v. 26, 2019.
- Development of GROMOS-Compatible Parameter Set for Simulations of Chalcones and Flavonoids ARANTES, P. R.; POLÊTO, M. D.; JOHN, E. B. O.; PEDEBOS, C.; GRISCI, B. I.; DORN, M.; VERLI, H. The Journal of Physical Chemistry B, v. 123, p. 994-1008, 2019.
- Neuroevolution as a tool for microarray gene expression pattern identification in cancer research GRISCI, B.; FELTES, B. C.; DORN, M. Journal of Biomedical Informatics, v. 89, p. 122-133, 2019.
- A Memetic Algorithm Based on an NSGA-II Scheme for Phylogenetic Tree Inference CID-VILLALOBOS, M.; DORN, M.; LIGABUE-BRAUN, R.; INOSTROZA-PONTA, M. IEEE Transactions on Evolutionary Computation, v. 23, p. 776-787, 2019.
- Architects meets Repairers: The interplay between homeobox genes and DNA repair FELTES, B. C. DNA Repair, v. 73, p. 34-48, 2019.
- A biased random key genetic algorithm for the protein–ligand docking problem LEONHART, P. F.; SPIELER, E.; LIGABUE-BRAUN, R.; DORN, M. Soft Computing, v. 23, p. 4155-4176, 2019.
2018
- Perspectives and applications of machine learning for evolutionary developmental biology FELTES, B. C.; GRISCI, B. I.; POLONI, J. F.; DORN, M. Molecular Omics, v. 14, p. 289-306, 2018.
- A multi-objective gene clustering algorithm guided by apriori biological knowledge with intensification and diversification strategies PARRAGA-ALAVA, J.; DORN, M.; INOSTROZA-PONTA, M. BioData Mining, v. 11, p. 1-22, 2018.
- Aromatic Rings Commonly Used in Medicinal Chemistry: Force Fields Comparison and Interactions With Water Toward the Design of New Chemical Entities POLÊTO, M. D.; RUSU, V. H.; GRISCI, B. I.; DORN, M.; LINS, R. D.; VERLI, H. Frontiers in Pharmacology, v. 9, p. 1-20, 2018.
- Everyone Is a Protagonist: Residue Conformational Preferences in High-Resolution Protein Structures LIGABUE-BRAUN, R.; BORGUESAN, B.; VERLI, H.; KRAUSE, M. J.; DORN, M. Journal of Computational Biology, v. 25, p. 451-465, 2018.
- Three-dimensional protein structure prediction based on memetic algorithms CORRÊA, L. L.; BORGUESAN, B.; KRAUSE, M. J.; DORN, M. Computers & Operations Research, v. 91, p. 160-177, 2018.
- A Memetic Algorithm for 3D Protein Structure Prediction Problem CORRÊA, L.; BORGUESAN, B.; FARFÁN, C.; INOSTROZA-PONTA, M.; DORN, M. IEEE/ACM Transactions on Computational Biology and Bioinformatics, v. 15, p. 690-704, 2018.
2017
- NEAT-FLEX: Predicting the conformational flexibility of amino acids using neuroevolution of augmenting topologies GRISCI, B.; DORN, M. Journal of Bioinformatics and Computational Biology, v. 15, p. 1750009, 2017.
- NIAS-Server: Neighbors Influence of Amino acids and Secondary Structures in Protein BORGUESAN, B.; INOSTROZA-PONTA, M.; DORN, M. Journal of Computational Biology, v. 24, p. 255-265, 2017.
2014
- Three-dimensional protein structure prediction: Methods and computational strategies DORN, M.; SILVA, M. B.; BURIOL, L. S.; LAMB, L. C. Computational Biology and Chemistry, v. 53, p. 251-276, 2014.
- MOIRAE: A computational strategy to extract and represent structural information from experimental protein templates DORN, M.; BURIOL, L. S.; LAMB, L. C. Soft Computing, v. 18, p. 773-795, 2014.
2013
- An interval-based algorithm to represent conformational states of experimentally determined polypeptide templates and fast prediction of approximated 3D protein structures DORN, M.; SOUZE, O. N. International Journal of Bioinformatics Research and Applications (IJBRA), v. 9, p. 462-486, 2013.
- A cluster-DEE-based strategy to empower protein design ANDRADES, R. K.; DORN, M.; FARENZENA, D. S.; LAMB, L. C. Expert Systems with Applications, v. 40, p. 5210-5218, 2013.
- A molecular dynamics and knowledge-based computational strategy to predict native-like structures of polypeptides DORN, M.; BURIOL, L. S.; LAMB, L. C. Expert Systems with Applications, v. 40, p. 698-706, 2013.
Laboratory Facilities
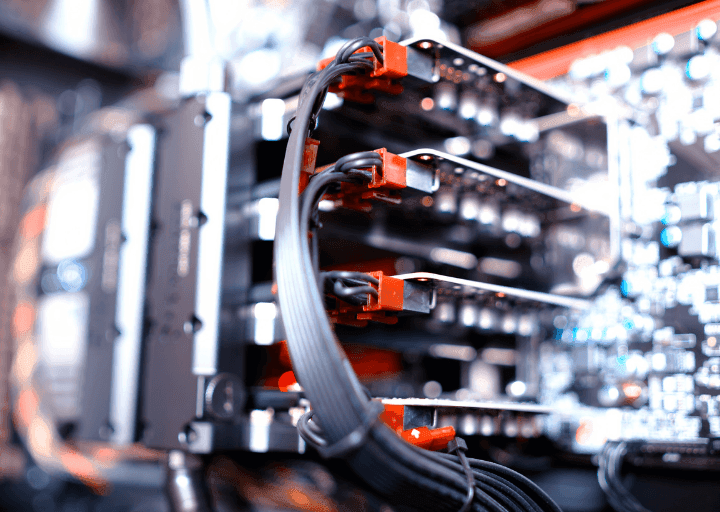
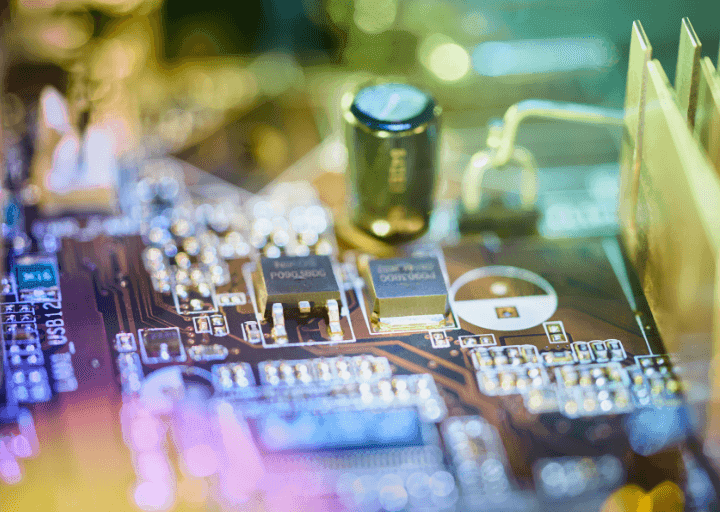
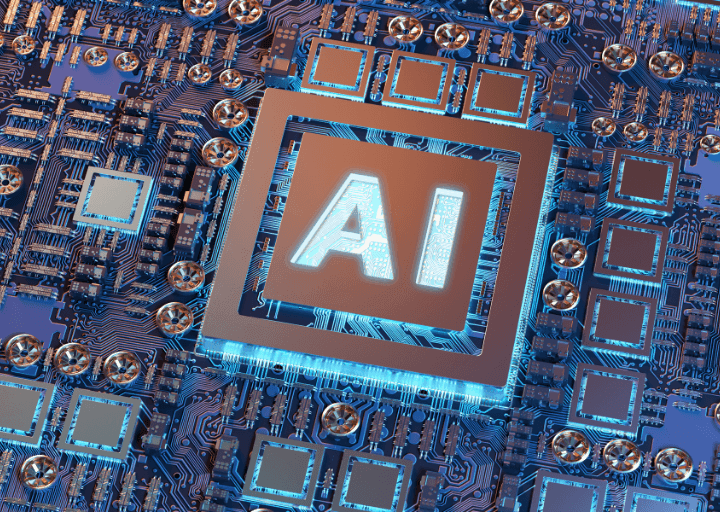
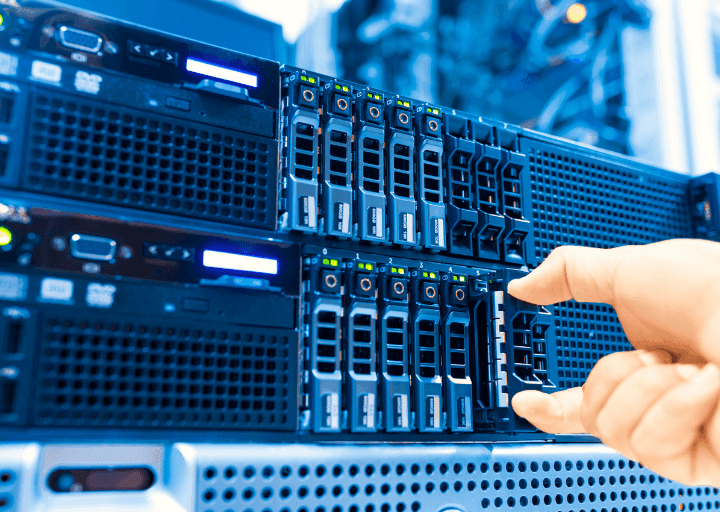
Scientific discoveries are closely linked to technological development. The Structural Bioinformatics and Computational Laboratory maintains and constantly expands cutting-edge facilities that enable students and scientists to carry out their research. The SBCB Lab also has access to international High Performance Computing infrastructure through cooperation projects with France, Chile, and Germany. The SBCB Lab is also member of the National Research Infrastructure Platform MCTI.
-
SBCB Server - Jupyter Lab
Jupyter enables interactive computing and is an alternative to accessing High-Performance Computing resources via SSH. It allows different programming languages and runtimes to be used within a web-based environment. While the front end runs in the browser on the client, the commands are executed on the remote systems. The SBCB Jupyter platform runs on 48 cores (Intel Xeon E5-2650V4), 768 GB memory, 5120 CUDA cores / 640 Tensor Core (Titan V GPU), and 200 TB of workspace. A detailed documentation of the Jupyter project can be found at https://jupyter.readthedocs.io.
-
SBCB Server - RStudio Server
RStudio Server enables a browser-based interface to R running in the remote server, bringing the power and productivity of the RStudio IDE to server-based deployments of R. The SBCB RStudio Server runs on 64 cores (Intel Xeon Silver 4216), 1 TB of Memory, and 200 TB of workspace. A detailed documentation of the RStudio Server project can be found at https://docs.posit.co/ide/server-pro/1.1.463.
Hardware Overview
-
Computing node 00 - sbcbserv00 - HP Proliant Server ML350E G8
Intel Xeon E5-2407, 2 CPUs, 2.2Ghz, 8 cores, 48G, 10TB. TESLA nvidia K40. 2880 CUDA core, 12 GB GDDR5, 288 GB/sec, 4.29 Tflops. Thanks to the NVIDIA Hardware Grant Program.
-
Computing node 01 - sbcbserv01 - IBM X3650 M5 Server
Intel Xeon E5-2650V4 30 MB, 2 CPUs, 2.2 Ghz, 48 threads - 768 GB DDR4 2400mhz RDIMM - HDD 4 TB SAS. Titan V GPU: Volta architecture, 5120 CUDA cores, 640 Tensor Cores, 12 GB HBM2, 652.8 GB/s Memory Bandwidth, 14,9 Tflops FP32, 110 Tflops ML. Thanks to the NVIDIA Hardware Grant Program.
-
Computing node 02 - sbcbserv02 - IBM X3650 M5 Server
Intel Xeon E5-2650V4 30 MB, 2 CPUs, 2.2 Ghz, 48 threads - 304 GB DDR4 2400mhz RDIMM - HDD 8TB SAS. Titan Xp GPU: Pascal architecture, 3840 CUDA core, 12 GB GDDR5X, 547.7 GB/s Memory Bandwidth, 10.97 TFLOPS Tflops FP32. Thanks to the NVIDIA Hardware Grant Program.
-
Computing node 03 - sbcbserv03 - IBM Lenovo SR650
Intel Xeon Silver 4216, 2 CPUs, 2.1Ghz, 64 threads - 1 TB DDR4 2933mhz RDIMM - HDD 12TB SAS. Titan X 3584 CUDA core, 12 GB GDDR5X, 480 GB/sec Memory Bandwidth, 11 Tflops. Thanks to the NVIDIA Hardware Grant Program.
-
Computing node 04 - sbcbserv04 - SILIX Server
AMD EPYC 7453, 2 CPUs, 3.45Ghz, 112 threads - 1 TB DDR4 3200mhz RDIMM - HDD 3TB SSD. 2 x Quadro Ampere RTX A5500, 24GB GDDR6 with ECC, 10.240 CUDA Core (34.1 TFLOPS), 320 Tensor Cores (272.8 TFLOPS), 80 RT Cores (66.6 TFLOPS), 768GB/sec Memory Bandwidth, CUDA NVLINK. Total GPU System with CUDA NVLINK: 20.480 CUDA Core, 640 Tensor Core, 160 RT Core, 48GB GDDR6.
-
2 x HP ProLiant Server ML310E G8
Intel Xeon E3-1220V2, 1 CPU, 3.1Ghz, 4 cores, 16GB, 500GB.
-
Data node 00 - sbcbdata00 - Lenovo ThinkSystem DE2000H Hybrid Storage Array
200 TB Lenovo ThinkSystem DE Series.
-
Data node 01 - sbcbdata01 - Storage Asustor Lockerstor8
144 TB Asustor Lockerstor. Storage NAS Asustor AS6508T (Quad Core 2.1GHz/8GB DDR4/10GbE/USB3.2/8)
-
Data node 02 - sbcbdata02 - TS-h1886XU-RP R2 Qnap
320 TB TS-h1886XU-RP R2 QNAP (AMD Ryzen 7 7000 32 GB DDR 5 10GbE).
-
Data node 03 - sbcbdata03 - TS-1673AU-RP - Qnap
320 TB TS-1673AU-RP QNAP (AMD Ryzen Quad-Core, 16 GB UDIMM DDR4 ).
External Infrastructure
-
Computing node Dayhof - Center for Biotechnology - UFRGS - Brazil
Computing node Dayhoff - Center of Biotechnology - Supermicro 4023S-TRT AMD EPYC 7502, 2 CPUs, 2.5Ghz, 128 threads - 512 GB DDR4 ECC - HDD 50TB. NVIDIA Tesla T4: Turing architecture, 2560 CUDA core, 320 Tensor Cores, 16 GB GDDR6, 320+ GB/s Total Memory Bandwidth, 254.4.
-
Horeka Green - Steinbuch Centre for Computing - Karlsruhe Institute of Technology - Germany
Through the cooperation with the Karlsruhe Institute of Technology (Germany), the Laboratory have access to the Horeka-Green supercomputer. It can provide a computing power of more than 17 PetaFLOPS or 17 quadrillion computing operations per second, which corresponds to the performance of more than 150,000 laptops. HoreKa is an innovative hybrid system with nearly 60,000 Intel processor cores, more than 220 terabytes of main memory and 668 NVDIA A100 GPUs.