DSBA
MCTI/CNPq/CT-Biotec nº 30/2022
Data Science for Biotechnology Applications
Solving large-scale challenges using explainable machine learning, metaheuristics, and high-performance computing
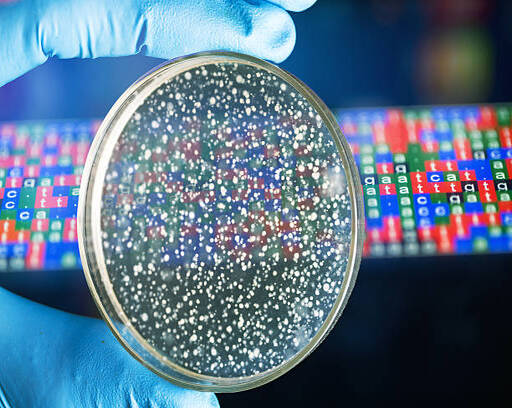
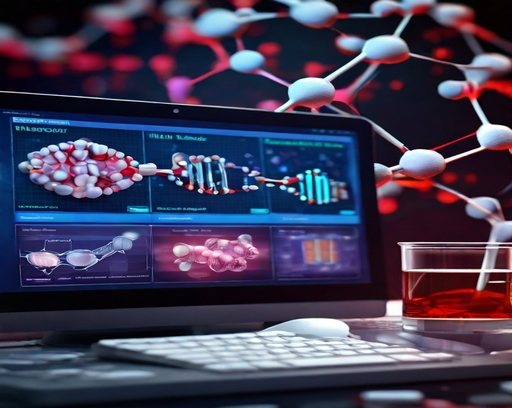
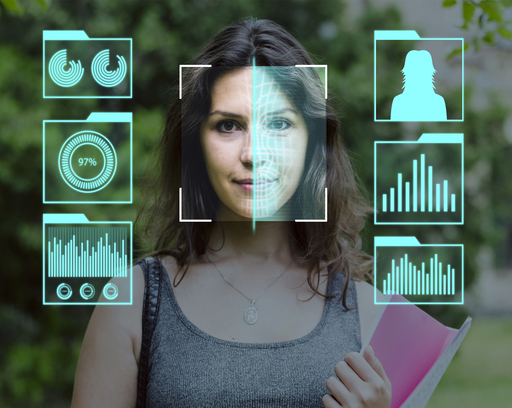
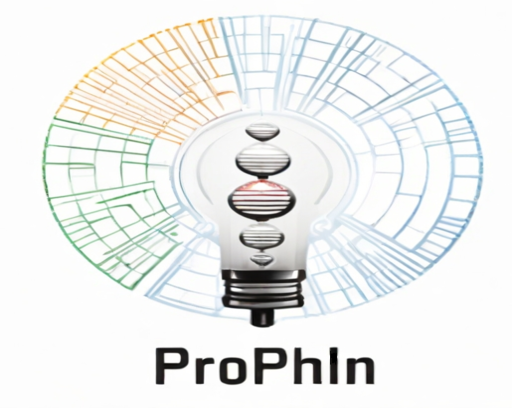
This project aims to develop new bioinformatics tools based on Machine Learning methods (supervised and unsupervised), heuristic search methods, and high-performance computing to explore high-dimensional data in problems of scientific and economic interest in the area of human and animal health. We will develop: (i) algorithms based on adaptive and multiobjective metaheuristics; (ii) multimodal metaheuristics; (iii) time series-based metaheuristics; (iv) combinatorial optimization; (v) interpretable machine learning methods; (vi) algorithms for feature extraction and selection; and (vii) combination of interpretability methods aiming at building general-purpose strategies that contribute to the analysis of large data with complex structure...
Animal Health
The use of bioinformatics tools in identifying molecular profiles of bacteria enables a precise and efficient approach to disease diagnosis. Furthermore, it fosters a deeper understanding of bacterial genetic diversity and facilitates well-informed clinical decision-making. In the field of animal health, researchers focus on studying bacteria of the genus Brucella, which cause a disease known as brucellosis. This disease, also called Malta fever or undulant fever, affects a wide range of mammals, exhibiting zoonotic and cosmopolitan characteristics and posing a significant risk to public health with substantial economic losses. Brucellosis can cause various symptoms, ranging from cold-like signs to complications in the nervous system, musculoskeletal system, and heart. In canines (affected by B. canis), nonspecific signs are observed, like those in humans, but reproductive failures and joint issues related to this bacterium are commonly diagnosed. Due to the diversity of clinical signs, diagnosing brucellosis in humans and animals presents a significant challenge, with underdiagnosis contributing to the spread of infection. Despite this, few genomic studies with different strains of B. canis have been developed so far. In this regard, there is a demand for more information, such as virulence factors, antimicrobial resistance genes, and the evolutionary profile of the pathogen, which can greatly contribute to decision-making in government responses to public health, as well as in storing and comparing data about this agent.
In the experimental front of this project, team members recently sequenced 20 B. canis genomes using two sequencing technologies (for obtaining short reads and long reads), which will contribute to the data used in solving this biological problem, along with 60 public genomes of B. canis and 160 public genomes of B. suis. This data will be analyzed by the computational tools developed in this proposal to identify species-specific genetic variations to serve as diagnostic markers for brucellosis. Interpretable machine learning algorithms will be employed to create a genotypic profile of virulent strains and differentiate them between species based on their phenotypic differences and antimicrobial susceptibility profiles.
Researchers
- Dr. Maria Cátira Bortolini IBIO/DeGen/UFRGS
- Dr. Bruno Iochins Grisci CBiot/INF/UFRGS
- Dr. Franciele Maboni Siqueira CBiot/FAVET/UFRGS
- Dr. Hugo Verli CBiot/IBIO/UFRGS
- Dr. Juliana Silva Bernardes LCQB/UPMC
- Dr. Manuel Villalobos-Cid DIINF/USACH
- Dr. Mario Inostroza-Ponta DIINF/USACH
Graduate Students/Collaborators
- Cauê Scotti Luciano Rocha ITI - EC/UFRGS
- Lorenzo C. C. Novo ITI - BTC/UFRGS
Scholarship Students
- Cauê Scotti Luciano Rocha ITI - EC/UFRGS
- Lorenzo C. C. Novo ITI - BTC/UFRGS
Publications
- NIAS-Server 2.0: A versatile complementary tool for structural biology studies FELTES, B. C.; PINTO, E. S. M.; MANGINI, A. T.; DORN, M. Journal of computational Chemistry, v. 44, p. 1610-1623, 2023.
- Meta-analyses of host metagenomes from colorectal cancer patients reveal strong relationship between colorectal cancer-associated species ESCALONA, M. A. R.; POLONI, J. F.; KRAUSE, M. J.; DORN, M. Molecular Omics, v. 19, p. 429-444, 2023.
- The nucleotide excision repair proteins through the lens of molecular dynamics simulations PINTO, E. S. M.; KRAUSE, M. J.; DORN, M.; FELTES, B. C. DNA Repair, v. 127, p. 103510, 2023.
- Functional response of microbial communities in lab-controlled oil-contaminated marine sediment JUNIOR, R. A.; POLONI, J. F.; ESCALONA, M. A. R.; DORN, M. Molecular Omics, v. 19, p. 756-768, 2023.